TECHNOLOGIE
///
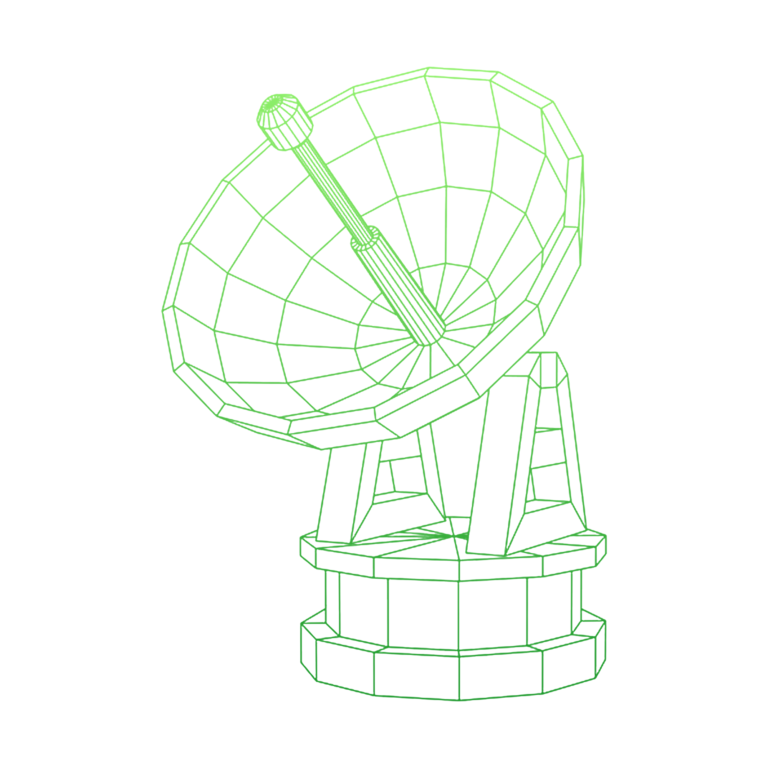
GENAUIGKEIT, ABDECKUNG UND MEHRWERT
Genauigkeit
Kayrros-Wissenschaftler haben zahlreiche Artikel in hochrangigen, von Experten begutachteten Fachzeitschriften verfasst. Dazu gehören:
Globale Bewertung der Methan-Ultra-Emittenten aus Öl und Gas. Wissenschaft.
Globale Muster der täglichen CO²-Emissionsreduzierung im ersten Jahr von COVID-19. Nature Geoscience.
Vergleich der in den UNFCCC-Inventaren angegebenen nationalen Treibhausgasbudgets mit atmosphärischen Inversionen.
Digitales Twinning aller Wald- und Nicht-Waldbäume auf nationaler Ebene durch Deep Learning.
Proceedings of the National Academy of Sciences.
Globale Verfolgung und Quantifizierung von Methanaustritten aus Öl- und Gasvorkommen anhand von wiederkehrenden Sentinel-2-Bildern.
Umweltwissenschaft und -technologie
EU-Kohlenstoffemissionen aus regulierten Sektoren: Tägliche Kayrros-Messungen vs. verzögerte offizielle jährliche Schätzungen
Kumulative Kayrros-Patentanmeldungen
Eine Liste der Veröffentlichungen, die unsere Ergebnisse zitieren, finden Sie
Kayrros arbeitet mit führenden Forschungseinrichtungen auf der ganzen Welt zusammen und hat zahlreiche technologische Patente angemeldet.
Die echtzeitnahen Daten von Kayrros haben sich stets als äußerst genaue Vorläufer der offiziellen Datenveröffentlichungen erwiesen, wann immer sie verfügbar sind.
So haben beispielsweise die täglichen, nahezu in Echtzeit durchgeführten Messungen der europäischen Kohlenstoffemissionen von Kayrros in zwei aufeinander folgenden Jahren mit einer Genauigkeit von mehr als 98,5 % mit den verzögerten Jahresdaten der EU übereingestimmt.
Abdeckung
Kayrros ist ein One-Stop-Shop für alle wichtigen Klimamessungen
Kayrros hat alle materiellen Güter, die auf globaler Ebene am wichtigsten sind, kartiert und misst alle Parameter, die für die Energiewende relevant sind, um die beste und umfassendste Erfassung von Energie und Umwelt zu gewährleisten.
Bei der Verarbeitung und Analyse von Satellitenbildern nutzt Kayrros eine schnell wachsende proprietäre Datenbank mit mehr als 3 Millionen physischen Assets, um alle Dimensionen der Energiewende abzudecken.
Kayrros misst neue Produktionskapazitäten, Produktionsvolumina, -ströme, -bestände, Zwischen- und Endverbrauch sowie die damit verbundenen Treibhausgasemissionen und Schadstoffe für alle Formen von Energie und damit verbundenen Materialien. Kayrros verfolgt auch das Wachstum der Energieinfrastruktur und modelliert und misst physische Risiken und Schäden im Zusammenhang mit dem Klimawandel
.
Tägliche Messungen von Dutzenden von Parametern und Millionen von Vermögenswerten schaffen die bestmögliche unabhängige Berichterstattung über Energie und Umwelt.
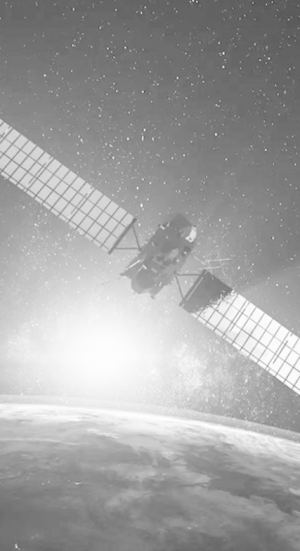
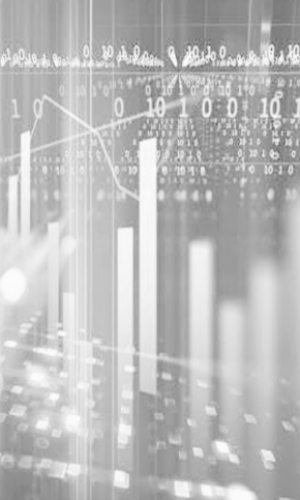
Mehrwert
///
AUSZEICHNUNGEN
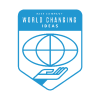
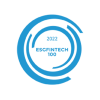
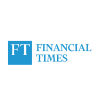
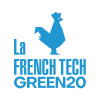
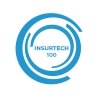
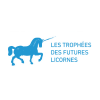
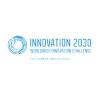
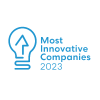
///
RECENT POSTS
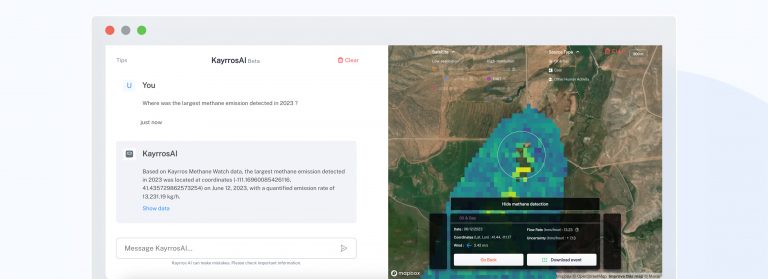
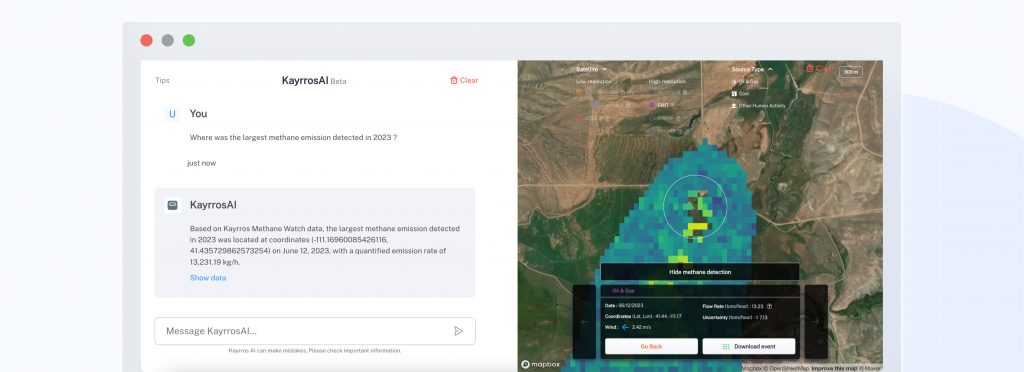
Kayrros releases KayrrosAI, first-of-its-kind methane-focused RAG LLM at COP29
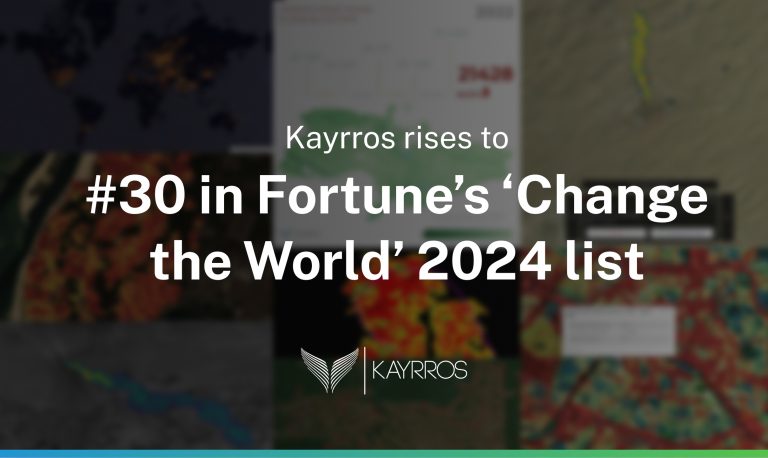
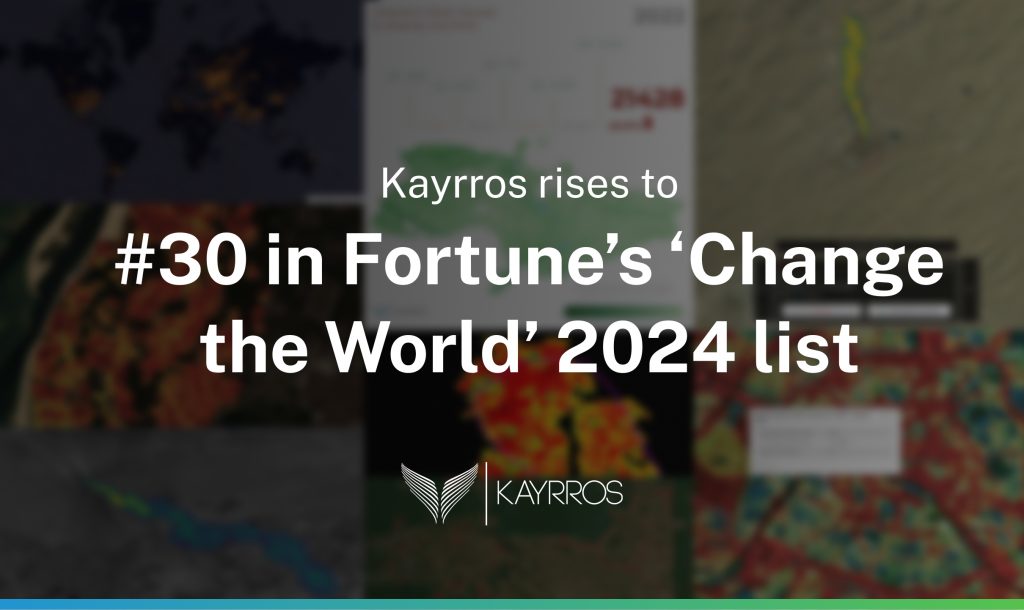
Kayrros rises to #30 in Fortune’s ‘Change the World’ 2024 list
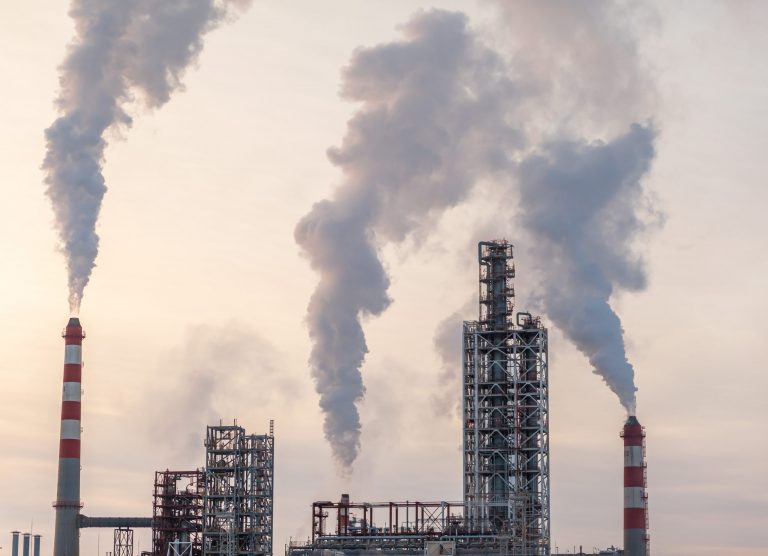
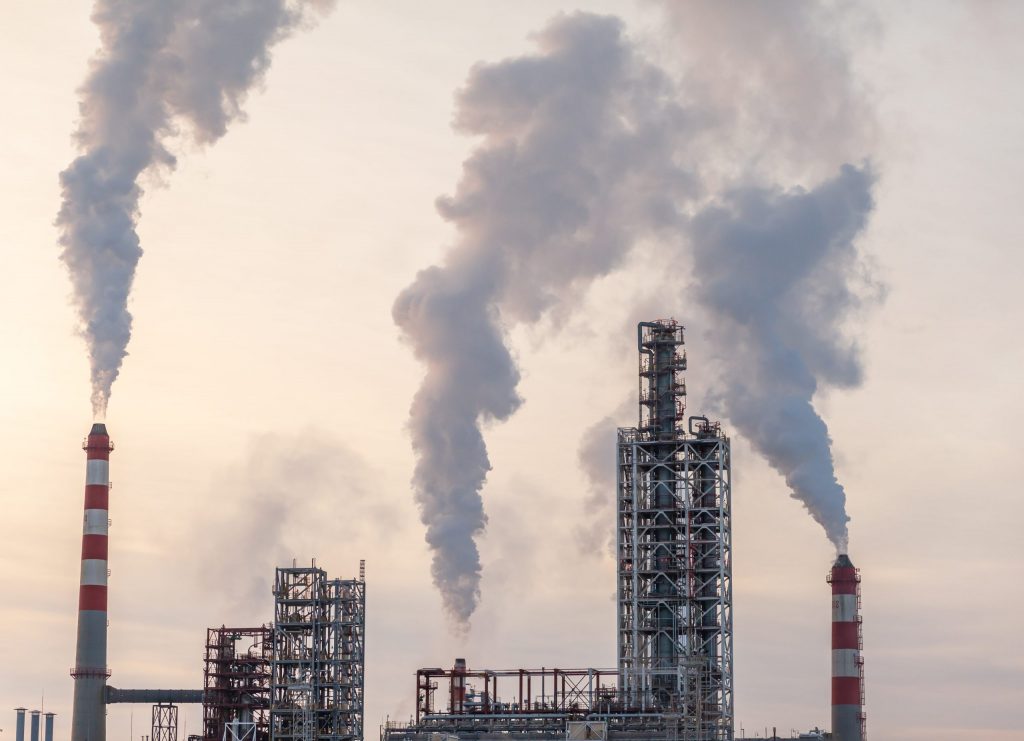
The Global Methane Pledge, Three Years On: Partial Progress Report